Media
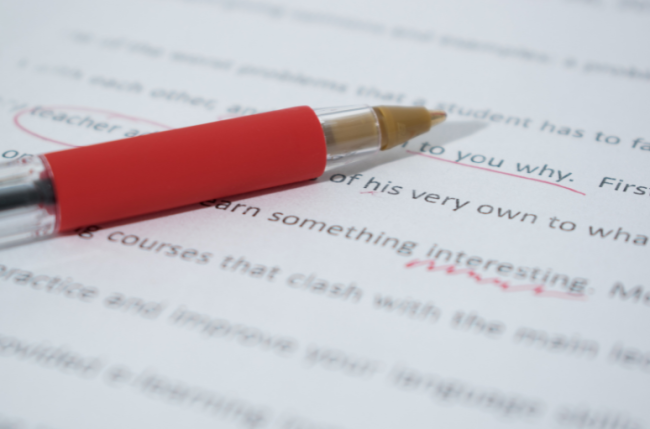
Stop with the “native speaker” writing “advice”
Inside Higher Ed, August 18, 2023Online
URL: https://www.insidehighered.com/opinion/views/2023/08/18/stop-native-speaker-writing-advice-opinion
We need to stop telling our students to have their essays checked by a native English speaker. It doesn’t help them, sometimes harms them, and perpetuates a false narrative about writing and language.
Measuring the non-existent: validity before measurement
by Kino Zhao
Published by Philosophy of Science (journal at Cambridge University Press)
February 17, 2023
This article examines the role existence plays in measurement validity. I argue that existing popular theories of measurement and of validity follow a correspondence framework, which starts by assuming that an entity exists in the real world with certain properties that allow it to be measurable. Drawing on literature from the sociology of measurement, I show that the correspondence framework faces several theoretical and practical challenges. I suggest the validity-first framework of measurement, which starts with a practice-based validation process as the basis for a measurement theory and only posits objective existence when it is scientifically useful to do so.
Sample Representation in the Social Sciences
by Kino Zhao
Published by Synthese (journal at Springer)
August 13, 2023
The social sciences face a problem of sample non-representation, where the majority of samples consist of undergraduate students from Euro-American institutions. The problem has been identified for decades with little trend of improvement. In this paper, I trace the history of sampling theory. The dominant framework, called the design-based approach, takes random sampling as the gold standard. The idea is that a sampling procedure that is maximally uninformative prevents samplers from introducing arbitrary bias, thus preserving sample representation. I show how this framework, while good in theory, faces many challenges in application. Instead, I advocate for an alternative framework, called the model-based approach to sampling, where representative samples are those balanced in composition, however they were drawn. I argue that the model-based framework is more appropriate in the social sciences because it allows for systematic assessment of imperfect samples and methodical improvement in resource-limited scientific contexts. I end with practical proposals of improving sample quality in the social sciences.
URL: https://link.springer.com/article/10.1007/s11229-020-02621-3
Biography
Dr. Kino Zhao completed her PhD in philosophy. She is an assistant professor at Simon Fraser University, and publishes on topics in the philosophy of science and of statistics, with a special focus on data-based reasoning in the social sciences.